The Need for Recategorized Video Game Labels: A Quantitative Approach
by Richard J. Simonson, Joseph R. Keebler, Shawn DohertyAbstract
Prior research has suggested that video game genre labels are an ineffective method of communicating a game's experience. Our investigation serves to provide a quantitative means of assessing experience communication effectiveness. We assessed game similarities by their associated game genre labels. The ratio between the small number of available developer-generated labels and the number of games led to too few labels to effectively delineate between similar and dissimilar games. The ratio between the large number of user-generated labels and the number of games led to too many labels to effectively cluster similar games with one another. However, games that had matching genres (e.g., direct sequels to each other) led to direct clustering. The result from this analysis provides evidence that this novel method of assessing games' communication experience works as intended and may be used by future research to assess the effectiveness of alternative categorization systems.
Keywords: Video Game Genre, Categorization, Multidimensional Scaling, Game Similarity, Clustering
Introduction
Video game genre labeling systems are a set of words or phrases that attempt to describe the experience a game delivers (e.g., action, adventure, racing, sports) to its potential user base. This system, which is currently in place and used by nearly all game developers, sellers and online libraries, is often described as ineffective at informing said users about an expected experience for most modern games (Clarke et al., 2017; Doherty et al., 2018). This is, mainly, because the system was designed at a time in which games had fewer mechanics players could interact with. Unfortunately, the system did not appropriately adapt with newer generations of games that had more complex interactivity. The consequence of this limited evolution of game classification is illuminated in games such as Super-Mario Bros and Grand Theft Auto, which receive the action tag from multiple game libraries (Clarke et al., 2017). Few methods, predominately game recommendation systems, currently exist that are designed to suggest games based on various factors, such as game labels or whether particular games are present in popular libraries. Prior work has also provided tools and analyses that seek to identify the relationship between games so that users can search for games based off of desired experiences or other needs. For example, Ryan et al. (2015) created a toolset that allows users to find games they would otherwise be unfamiliar with based off how they are described using a natural language processing approach. Additionally, Li & Zhang (2020) developed a network analysis relating user-generated Steam tags to further understand the complex relationships they create. However, these methods were limited due to the challenge this paper is attempting to elucidate; there are no known or available methods that successfully seek to quantifiably measure the effectiveness, or lack thereof, of genre classification systems in communicating experience to a potential user. Thus, the purpose of this methodological investigation is to describe the challenge that occurs when current game labeling systems are used as classification and suggestion methods. This paper futher seeks to provide a means of quantifiably measuring the accuracy of this video game experience communication system.
The Challenge of Communicating Game Experience
Advances in both the technological capabilities of gaming devices and the concept of what a video game can be have contributed to complex open concept games. Such games might include Minecraft, which can be manipulated into nearly any type of genre based on the user’s play style. Additionally, exceedingly abstract games such as Museum of Simulation Technology use dimension-bending mechanics to shape the world around them. For example, the gameplay in Minecraft allows players to run massive roleplay servers, employ first-person gaming combat, build complex networks of machines, spelunk through dynamic cave systems and build massive structures such as complex city-like systems. Further, the game is moddable (i.e., users can modify the game code to change the gameplay experience), which allows one’s imagination to create any experience they like. To more effectively conceptualize a given game’s gameplay experience, many video game players rely on game reviews and word-of-mouth testimonials from other players. Further, many players tend to relate their experiences to other games to build a conceptualization based on past gaming experiences. A phrase from multiple video game reviews that exemplifies this describes the game Fallout 4 (developer genres: role-playing game) and Far Cry 3 (developer genres: action, adventure) to players as “like Skyrim (developer genres: role-playing game) with guns” (Ferrell-Wyman, 2015; Kovic, 2012). As shown by the included developer genres, these games are seemingly dissimilar from each other. Kovic (2012) describes them as very similar, where the only distinction between Skyrim and either Fallout 4 or Far Cry 3 is that a player has access to in-game guns. However, further incongruity between these games is suggested by the developers’ narrative descriptions, which paint entirely different pictures of each game that may not be garnered by either Kovic’s (2012) review or the developers’ suggested genres. The current video game genre system attempts to provide potential game users with a brief understanding of their game by relating the proposed experience to other games by genres such as action, adventure, platformer and many others. These suggested phrases attempt to inform a user that an action game may feel like other action games they have played. An example of the limitation of this current system are the vast differences between the popular Call Of Duty games and the Portal series. Multiple outlets use similar genre labels to describe these games including first-person, shooter and arcade where the only differentiator between these games is the inclusion of the puzzle genre for the Portal series (GameSpot, 2007; GameSpot, 2019; Metacritic 2007; Metacritic, 2019 Steam, 2007; Steam, 2007a). However, the experience and purpose of each game’s gameplay are completely different, as becomes evident when comparing their narratives. The first-person shooter Call Of Duty series offers a play experience described as “the most intense and cinematic action experience ever” with “an arsenal of advanced and powerful modern-day firepower” that “transports them to the most treacherous hotspots around the globe to take on a rogue enemy group threatening the world” (Steam, 2007). The first-person shooter Portal, on the other hand, is described as allowing players to “solve physical puzzles and challenges by opening portals to maneuvering objects, and themselves, through space” (2007a). The simplicity of genre labels between these two games advertise similar play experiences in that they share three out of four genre descriptive terms. However, more robust descriptions of game content provides imagery of completely different games. Players that rely on such labeling systems may be misguided when choosing their games.
A further challenge presented by the incongruites of the current labeling system can be seen in research communities that use video games as mediums for simulated environments. A plethora of sociotechnical research initiatives rely on video games to increase the cognitive fidelity of a simulated task due to their financial efficiency and accessibile, commercial off-the-shelf (COTS) status. For example, Hutchinson et al. (2016) investigated the use of action video games, specifically Call Of Duty, on reducing the Simon effect of visual stimulus and response times. Tannahill et al. (2012) also used Call Of Duty to review of how its mechanics and dynamics can facilitate learning. Similarly, Shute et al. (2015) employed Portal 2 and Luminosity to investigate their effects on participants' problem solving, spatial skills and persistence abilities. As indicated by their reasoning for using these games, researchers may rely on certain mechanics that can be described in terms of genre. However, if the labeling system is inaccurate at describing a game’s features, then successfully identifying a COTS that meets particular research needs may be impossible without word-of-mouth suggestions.
In an attempt to mitigate this challenge, prior research initiatives have attempted to provide a theoretically driven classification system of games primarily derived by their functions (Hunicke et al., 2004), deconstruct the approaches to semantically describing games from a cognitive and cultural standpoint (Vargas-Iglesias, 2020), or have attempted to provide evidence that current video game genre methods are ineffective at communicating game-play themes to potential players (Doherty et al., 2018; Clarke et al., 2015). However, no prior research has suggested or implemented a quantifiable means of measuring these ineffective systems. Thus, this investigation attempts to provide such a quantitative analysis. Our study utilized the popular online video game library Steam to gather the most comprehensive data available on video game labels, cluster the games based on attribute similarity (i.e., genre labels), and hierarchically cluster them into a visually meaningful format. The result of these efforts led to a spatial list of games, where each game was juxtaposed against other games with shared genre labels. We hypothesize that if the genre labeling system used by many game developers and libraries is effective enough at describing a game’s experience, then video games with similar experiences will be clustered together. Alternatively, if it is ineffective, then many video games will be clustered together that do not share similar descriptive experiences.
Method
Game Collection Source
Steam, a well-known online game library with millions of simultaneous users was used as our source to collect and catalog game using Scrapy (Zyte, 2019, Version 1.6) for Python. The reasoning behind this decision concerned a unique feature of Steam’s, wherein two different types of game labeling systems are used to describe a game’s potential experience. These two systems include a developer- and a user-based genre labeling schema. Further, Steam’s filtering system allowed us to sort the most popular and well-known games within Steam’s store to sample the highest quality list of the most played and most well-known games possible. These games’ widespread familiarity also helps convey our investigation’s results.
Sample Characteristics
The developer-based genre labeling system is a schema that is well-known and used throughout the gaming world. These genre labels are described and defined by game developers and designers, and are attached to the product at shipment. Table 1 provides information about the number of and types of labels included in the dataset. The “indie” genre was the most popular with 103 games, whereas “free to play” was the least popular and was subsequently excluded due to its sample of one game.
Labels |
# of Games |
Indie |
103 |
Action |
97 |
Simulation |
85 |
Adventure |
60 |
Strategy |
60 |
RPG |
49 |
Early Access |
36 |
Casual |
19 |
Massively Multiplayer |
18 |
Sports |
13 |
Racing |
10 |
Free to Play |
1 |
Table 1. Summary of Developer-Generated Genre Labels
The user-based genre labeling system is a schema that is unique to Steam. These genre labels are described and defined by players. While logged into Steam and viewing a game page, a user can submit a word or phrase that they feel describes an experience that the game provides. The most common user-submitted labels are then shown on the game page. Subsequently, users can provide words and phrases that are close synonyms to each other, which are not automatically rejected upon submission. Therefore, before aggregating the user-generated genre labels list, a synonym analysis was conducted to remove various repeated terms (e.g., “FPS” and “First Person Shooter”; “3D” and “3 Dimensional”). Additionally, any user-generated label with only one game was excluded. Following this data cleaning process, of the 265-user generated genre-labels extracted, 186 remained. The top five most and least common labels are presented in Table 2.
Labels |
# of Games |
Single player |
154 |
Action |
128 |
Multiplayer |
121 |
Indie |
117 |
Adventure |
104 |
Beautiful |
2 |
Beat’em up |
2 |
Based on a Novel |
2 |
Action-Adventure |
2 |
3D Platformer |
2 |
Table 2. Summary of User-Generated Labels.
Procedure
208 of the bestselling, safe-for-work (i.e., games that were not locked behind steams age verification) games as of 23 February 2019 were mined from the Steam Webstore using Scrapy for Python. We extracted each game’s meta-information from the scraped data, which included a game’s title, its developer-generated genre labels and its user-generated genre labels. Only the most common user-generated genre labels were used, as those are the only labels Steam displays on game pages. We then separated the two labeling schemas and categorized them into rows with their associated games in columns. Following this step, we binary-coded the collected games based on their included genres (Table 3).
Game |
Casual |
RPG |
Simulation |
Uno |
1 |
0 |
0 |
Star Trek: Bridge Crew |
1 |
1 |
1 |
Rocksmith 2014 Edition - Remastered |
1 |
0 |
1 |
Table 3. Example of binary coded games and genre labels.
Variables and Design
We used a similarity-based clustering algorithm to measure and compare video games from Steam for both developer and user-generated genre labels. This required using our main variable, the (dis)similarity measurement. (Dis)similarity measurement techniques attempt to quantify the distance between two points in some space, which provides a number that defines how closely related to each other they are. For example, this technique can be applied to states or provinces within a country. In the United States, specifically, one could determine how spatially similar each state is by placing a state/coordinate matrix into the similarity algorithm. The result would be a matrix that describes how close each state is to one another. In this example, Florida and Georgia would be more, quantitatively, spatially similar than Florida and New York. However, as our dataset contains a binary matrix of feature (i.e., genre labels) inclusion and exclusion, a Euclidian (e.g., linear) based distance algorithm is not appropriate. Rather, we use the Jaccard distance index (Eq 1.) which is suitable for binary datasets (Choi, Cha, & Tappert, 2010).
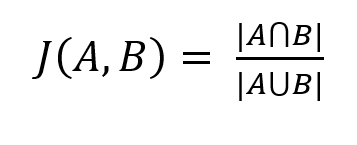
The Jaccard distance index is a metric that seeks to probabilistically relate two lists of features together. The resulting outcome of the index is a value between 0 and 1, where 1 describes two games that are 100 percent similar, or, are metrically the same, and 0 describes two games that are 0 percent similar, or completely dissimilar. When applied to a database of games and their associated features, the Jaccard index can transform the database into a matrix defining the distance between each game based on its included genres.
Analysis Approach
Following our distance calculation of video game (dis)similarities, we conducted a data reduction technique and factor loading process via stress minimization using majorization multi-dimensional scaling (SMACOF MDS). Similar to principal components analysis (PCA), but flexible with binary datasets, MDS reduces high dimensional data into multidimensional factors of related information (Hout et al., 2013). Where PCA reduces and combines data into factors based on correlations, MDS reduces and combines data based on their distances. Thus, we might expect games that share the same features (i.e., genre labels) to be loaded onto the same factor. We determined the number of clusters in the MDS analysis via a multi-faceted approach. First, we utilized a differential stress plot to determine, visually, which number of clusters resulted in the lowest net difference in stress between two dimensions. Stress is a measurement of goodness of fit for MDS and is calculated by the square-root difference between interpoint distances of items and the smoothed predicted distances of the distance index within the MDS (Wilkinson, 2002). The challenge with using stress as a method of goodness of fit, however, is that it is subject to the complexity of the data being implemented (Borg and Groenen, 2005, p. 48). If the data used is complex, has a large dimensionality, and/or the data generation was inconsistent and not based on a standardized approach, then higher levels of stress that maight otherwise be considered a poor fit would indicate a good fit. To combat this challenge, we next implemented normalized stress guided by our stress difference plot to increase the interpretability of the goodness of fit statistic. Normalized Stress is a transformation of the stress value that is interpreted like the statistic, where the value of normalized stress is equivalent to the amount of variance unaccounted for in the MDS model (Borg and Groenen, 2005, p. 249). We chose to guide this normalized stress with the stress differential plot, as there is no true cut-off for a good, normalized stress value. Rather, the smaller the value the better the model fit. However, normalized stress will become progressively smaller with every added dimension until the number of included dimensions equals the number of original data points. Using our stress differential plot provided us with an estimate of optimal, normalized stress based on the number of dimensions.
Visualizing the data’s similarity structures required using hierarchical clustering, as some of the genre label similarity matrices were still in a high-dimensional space after the data reduction analysis due to the high heterogeneity of genre labels. Hierarchical clustering is a visualization technique that serves to combine like items together from high-dimensional data. The visualization of hierarchical clustering analysis is a dendrogram that is defined by its branching hierarchical arms that describe the relationships between items. The furthest down branches (level 1) are the individual items, the second branch -- called clades (level 2) -- describes the similarity between each item where the closer the items are to each other, the more similar they are. The third branch (level 3) is the first layer of clusters. Items in clusters are more similar to each other than items in other clusters. This pattern can continue to an n number of clades and clusters depending on the complexity of the data being visualized. Further, clustering techniques applied after MDS analyses, such as hierarchical clustering, can provide an intuitive understanding of similarity structures in complex data. Such clustering techniques have been successfully utilized in prior high-dimensional research (Sireci, & Geisinger, 1992). The number of hierarchical clusters within this reduced dimensional data set was determined by Ward’s method, which measures cluster viability based on the within-cluster sum of squares error (Szekely & Rizzo, 2005). The final output of this analysis procedure is a dendrogram of games based on their similarities to one another as measured by their inclusion or exclusion of genre labels.
Results
Developer-Generated Labels
The stress plot indicated that a six-dimension solution was an optimal fit, which resulted in a stress value of .13 and a normalized stress of .002. The normalized stress suggests that .2 percent of the information clustered from the analysis is not accounted for. Visualization was conducted through hierarchical clustering, which resulted in a three clustered solution. Figure 1 provides a visualization of a subset of relationships between the included games, which can be interpreted as a proximal-similar relationship. Based on the subset we find games that are clustered in the same clade such as BeamNG: Drive and Species Artificial Life, Real Evolution share more features (10/11 features matched) than games featured in separate clades such as BeamNG: Drive and Project CARS 2 (9/11 features matched). These groupings provide evidence that this clustering technique is doing as it was hypothesized it would do. However, the lack of actual similarity between these games indicates a potential failure in the mechanism in which the labels were generated and are used. This full hierarchical relationship between games is available in Supplemental Figure 1.
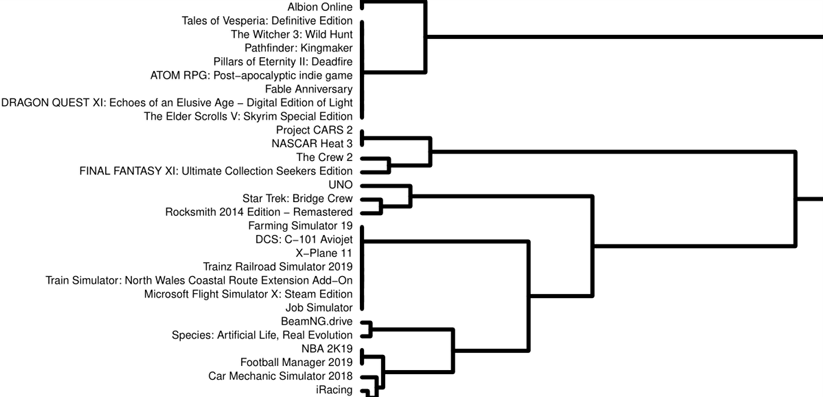
Figure 1. Subset of Developer-Generated Label Similarities. Click image to enlarge.
User-Generated Labels
The stress plot indicated an 11-dimension solution where .8 percent (stress = .091, normalized stress = .008) of the information from the data was not accounted for in the MDS. Similar to the developer-generated labels we find that games that are clustered in the same clade (Figure 2.) such as Total War: Warhammer and Total War: Warhammer II share more features (179/186 features matched) together than games featured in separate clades such as Total War: Warhammer and Grim Dawn (163/186 features matched). These groupings provide evidence that this clustering technique is effectively capturing the similarities of these games based on their genres. This full hierarchical relationship between games is available in Supplemental Figure 2.
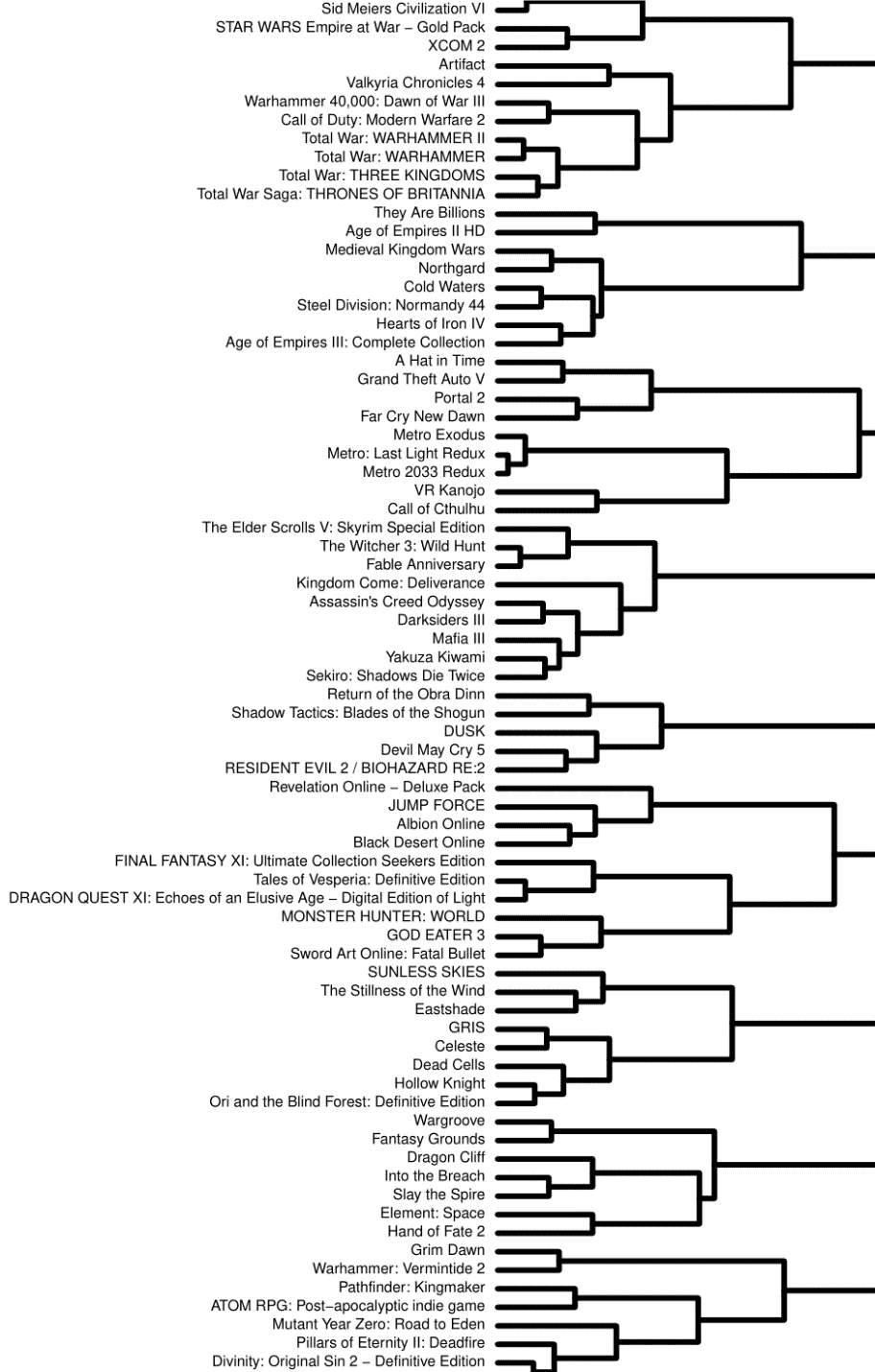
Figure 2. Subset of User-Generated Label Similarities. Click image to enlarge.
Discussion
Our application of this novel approach to quantitatively compare games based on their similarities provides evidence that 1) this novel approach may provide a quantitative assessment in determining if a video game experience communication system is correctly matching games with similar games, and 2) provides a quantitative method of confirming prior hypotheses that the current approach of communicating experience is ineffective.
First, the results of this approach show that this method is effective at clustering games to one another based on their provided genres. This is evident in a multitude of examples from our included dataset, but is exemplified in our results through the sequels such as the Total War series, which share the same genre labels and were all clustered together. We posit the significance of these finding lies in using quantifiable and output-driven methods as a means to appropriately group games with similar experiences without relying on subjective opinion. Future research should utilize this method to test the ability of previously suggested game experience communication methods, such as the cognitive approaches (c.f., categorization based on cognitive psychology or user experience, Boot et al., 2011; Feng et al., 2007; Green & Bavelier, 2007) and game design mechanics (e.g., MDA) (c.f., Hunicke et al, 2004; Phan et al, 2016 ; Wilson et al, 2009) to cluster similar games together. Additionally, we posit that if a system is tested and is shown to work, developers, users and researchers alike can rely on this clustering method or others that group games based on their proposed features without relying on extensive word-of-mouth reviews. Many potential solutions have been posited, including the use of alternative experiential communication methods (e.g., MDA, game user satisfaction, cognitive benefits), but we posit that improvements may also be made on the game genre labels themselves. Such improvements may be in assigning weights to genre labels or distinguishing between their purpose (i.e., mechanical, thematic, style).
Second, current video game experience labeling practices have been suggested to be ineffective (Doherty et al, 2018). We provide a quantitative comparative similarity analysis of video games based on the currently utilized schema in an attempt to assess whether or not a game's experience is being properly related to other games labeled similarly. Our results, under this current paradigm, suggest that developer-generated video game genre labels do not intuitively communicate gameplay experience. This evidence comes from our multidimensional scaled hierarchical clustering of video game similarities based on the inclusion of these developer-generated labels. Games that are closely clustered together, such as the evolution and life simulator Species: Artificial Life, Real Evolution, and the high-fidelity driving simulator BeamNG: Drive (10/11 features shared) offer completely different experiences to a player or potential player as suggested by the description of the games supplied by the developers themselves (Steam, 2018b; Steam, 2015). Additionally, 11 different labels were used to define 208 games. In doing so, a significant amount of information and comparative power is lost between each game, as on average, only 2.64 (SD = 1.46) labels are used to describe a game. Under this paradigm of using a small handful of industry-accepted genre labels, the system suffers from low variability and accuracy challenges wherein too few labels result in clusters of games that are experientially dissimilar to one another. An alternative to the developer-based system is the use of community-driven user-generated genre labels. Developers may often be limited by marketing strategies and other factors in fully developing a set of mechanics in which to describe gameplay experience in a compact and meaningful manner. Steam implemented a feature that possibly mitigates this challenge in a way where the player base of a game can contribute words or short phrases that may more effectively capture the complexities that are game experiences. Our results, however, show that this may still not be the case. While more information was captured that allowed for, possibly, a more accurate clustering of games, we still see games that completely differ in experience as described in their game descriptions -- such as the virtual reality personality simulator VR Kanojo (Steam, 2018c) and the first-person horror game Call of Cthulhu (Steam, 2018a) (166/186 features shared), which are supposedly similar based on their genre-labels. Further, 186 user-generated labels describe 208 games, where on average 15 labels (SD = 5.53) are used to describe a game. Conversely, the user-generated labels have too many unique labels, which may result in the artificial clustering of games.
Limitations
Multidimensional scaling and hierarchical clustering are both considered descriptive analyses. As such, no inferences can be made about the specific relationships from the resulting outputs of the model. In this sense, as we did not collect every game and its associated features on Steam, some of the unusual relationships present in the analysis may only have occurred because there was not a better-suited game in our collected sample to match them. This may have been present within the population of games we did not collect. Additionally, as MDS is a descriptive analysis, no power analysis or other sample-size determinants are available to use. Rather, we collected the number of games and features based on resource allocation. Thus, the main focus of this investigation was not to analyze the relationships between games, rather, it provides evidence that this novel method is effective at determining the relationship between said games based on the features used to describe them. Additionally, this analysis required including every genre label gathered, and considered two games that share a lack of features as equally similar as two games that share features. In other words, two games that do not include the horror genre and two games that do share the horror genre will have the same similarity score. However, this limitation may further highlight the challenge of describing a game's experience with the genre labeling method.
Conclusion
The purpose of video game genre labels is to inform potential users about various features and elements a game has so that they can form an a-priori idea of the experience the game elicits without investing time and money into the game. While these concepts heavily apply to the business of selling games and targeting a user base, they also apply to help researchers find games that fit their research and study needs. The resulting models provide evidence that this novel method successfully quantifiably groups games together based on provided descriptions of their features. This method should be applied in identifying, from an objectively mathematically driven perspective, new video game experience communication systems that more accurately communicate and describe the experience of modern and complex games.
Appendix: Supplemental Tables
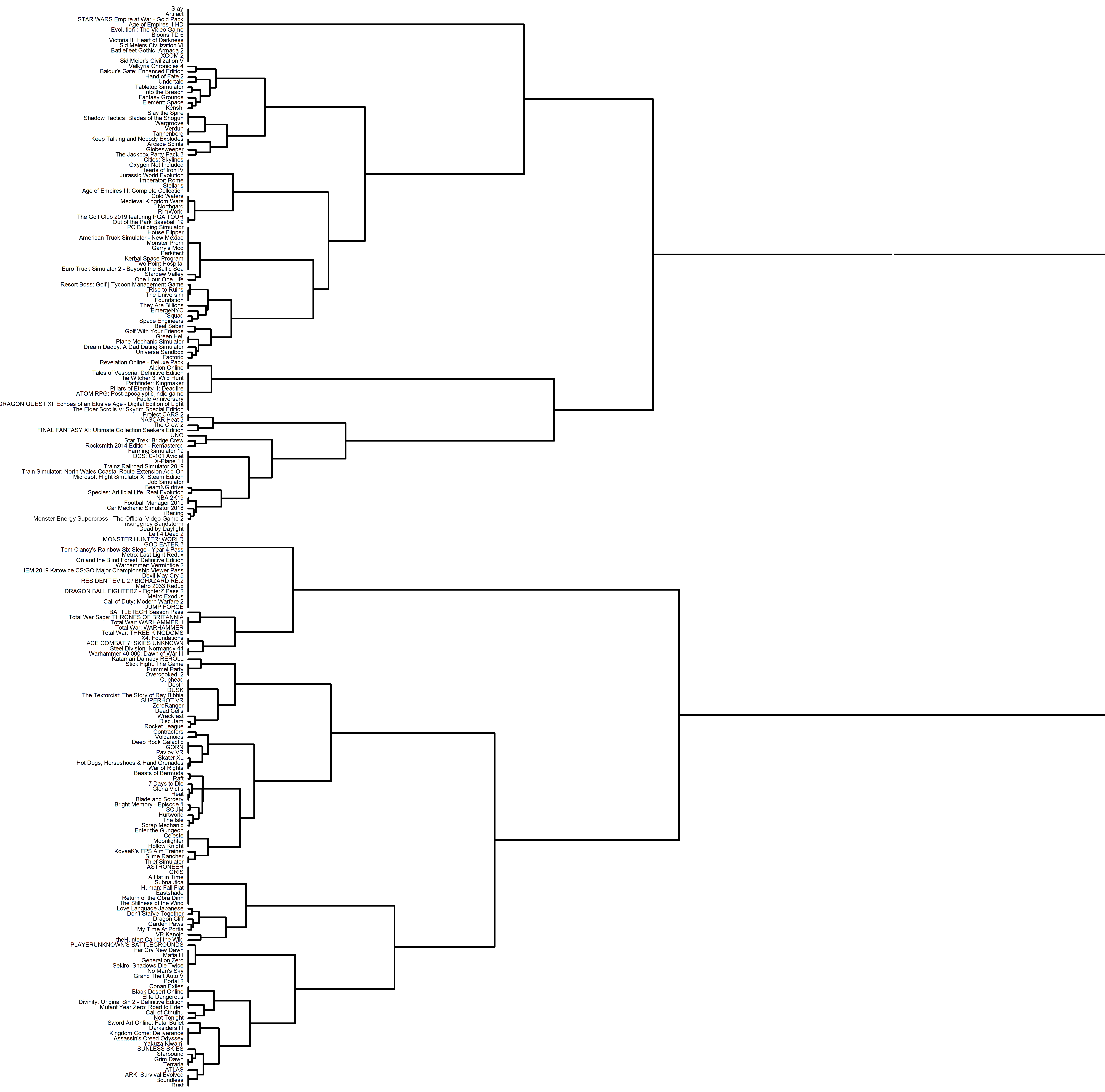
Supplemental Table 1. Click image to enlarge.
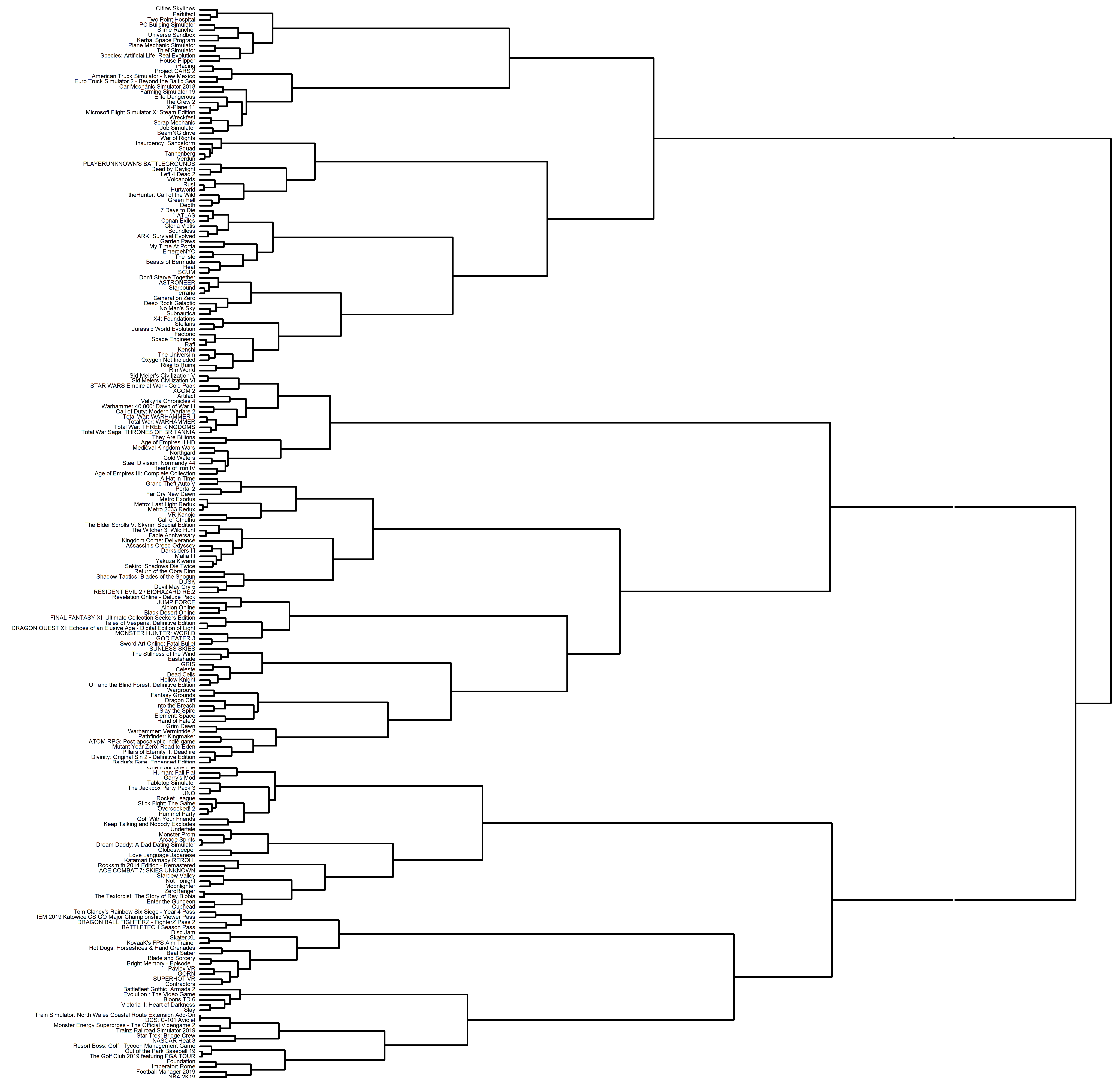
Supplemental Table 2. Click image to enlarge.
References
Boot, W. R., Blakely, D. P., & Simons, D. J. (2011). Do action video games improve perception and cognition?. Frontiers in psychology, 2, 226.
Borg, I. and Groenen, P. (2005). Modern multidimensional scaling. Springer.
Choi, S. S., Cha, S. H., & Tappert, C. C. (2010). A survey of binary similarity and distance measures. Journal of Systemics, Cybernetics and Informatics, 8(1), 43-48.
Clarke, R. I., Lee, J. H., & Clark, N. (2017). Why video game genres fail: A classificatory analysis. Games and Culture, 12(5), 445-465. https://doi.org/10.1177/1555412015591900
Doherty, S. M., Keebler, J. R., Davidson, S. S., Palmer, E. M., & Frederick, C. M. (2018). Recategorization of Video Game Genres. In Proceedings of the Human Factors and Ergonomics Society Annual Meeting, 62(1), 2099-2103. doi:10.1177/1541931218621473
Feng, J., Spence, I., & Pratt, J. (2007). Playing an action video game reduces gender differences in spatial cognition. Psychological Science, 18(10), 850-855.
Ferrell-Wyman, S. (2015, November 16). Fallout 4 review: Like Skyrim with guns. The Tacoma Ledger. http://thetacomaledger.com/2015/11/16/fallout-4-review-like-skyrim-with-guns/
GameSpot. (2007, October 10). Portal. https://www.gamespot.com/games/portal/
GameSpot. (2019, October 25). Call of duty: Modern warfare. https://www.gamespot.com/games/call-of-duty-modern-warfare/
Green, C. S., & Bavelier, D. (2007). Action-video-game experience alters the spatial resolution of vision. Psychological Science, 18(1), 88-94.
Hout, M.C., Papesh, M.H. and Goldinger, S.D. (2013). Multidimensional scaling. WIREs Cogn Sci, 4(1), 93-103. doi:10.1002/wcs.1203
Hunicke, R., LeBlanc, M., & Zubek, R. (2004). MDA: A formal approach to game design and game research. In Proceedings of the AAAI Workshop on Challenges in Game AI , 4(1), 1722.
Hutchinson, C. V., Barrett, D. J., Nitka, A., & Raynes, K. (2016). Action video game training reduces the Simon Effect. Psychonomic bulletin & review, 23(2), 587-592.
Shih, A. (n.d.). Museam of Simulation Technology | 2014 Student Entry | Independent Games Festival. Independent Games Festival. Retrieved November 1, 2022, from https://igf.com/museum-simulation-technology
Kovic, A. (2012). GamesCon. North Rhine-Westphalia.
Li, X., & Zhang, B. (2020, January). A preliminary network analysis on steam game tags: another way of understanding game genres. In Proceedings of the 23rd International Conference on Academic Mindtrek (pp. 65-73).
Metacritic. (2007, October 10). Portal. https://www.metacritic.com/game/pc/portal
Metacritic. (2019, October 25). Call of duty: Modern warfare. https://www.metacritic.com/game/playstation-4/call-of-duty-modern-warfare
Phan, M. H., Keebler, J. R., & Chaparro, B. S. (2016). The development and validation of the game user experience satisfaction scale (GUESS). The Journal of Human Factors and Ergonomics Society, 58(8), 1217-1247. doi:10.1177/0018720816669646
Ryan, J. O., Kaltman, E., Mateas, M., & Wardrip-Fruin, N. (2015, June 22-25). What we talk about when we talk about games: Bottom-up game studies using natural language processing [Conference paper]. Foundation of Digital Games 2015 Conference, Pacific Grove, CA, USA.
Shute, V. J., Ventura, M., & Ke, F. (2015). The power of play: The effects of Portal 2 and Lumosity on cognitive and noncognitive skills. Computers & education, 80, 58-67.
Sireci, S. G., & Geisinger, K. F. (1992). Analyzing test content using cluster analysis and multidimensional scaling. Applied Psychological Measurement, 16(1), 17-31.
Steam. (2007). Call of duty 4: Modern warfare. https://store.steampowered.com/app/7940/Call_of_Duty_4_Modern_Warfare/
Steam. (2007a). Portal. https://store.steampowered.com/app/400/Portal/
Steam. (2015). BeamNG.drive. https://store.steampowered.com/app/284160/BeamNGdrive/
Steam. (2018a). Call of cthulhu. https://store.steampowered.com/app/399810/Call_of_Cthulhu/
Steam. (2018b). Species: artificial life, real evolution. https://store.steampowered.com/app/774541/Species_Artificial_Life_Real_Evolution/
Steam. (2018c). VR kanojo. https://store.steampowered.com/app/751440/VR_Kanojo__VR/
Szekely, G. J., & Rizzo, M. L. (2005). Hierarchical clustering via joint between-within distances: Extending Ward's minimum variance method. Journal of classification, 22(2), 151-183.
Tannahill, N., Tissington, P., & Senior, C. (2012). Video games and higher education: what can “Call of Duty” teach our students?. Frontiers in psychology, 3, 210.
Vargas-Iglesias, J. J. (2020). Making sense of genre: The logic of video game genre organization. Games and Culture, 15(2), 158-178.
Wilkinson L. (2002). Multidimensional scaling. In Systat 10.2 Statistics II (pp. 119-145). Systat.
Wilson, K. A., Bedwell, W. L., Lazzara, E. H., Salas, E., Burke, C. S., Estock, J. L., ... & Conkey, C. (2009). Relationships between game attributes and learning outcomes: Review and research proposals. Simulation & gaming, 40(2), 217-266.
Zyte. (2019). Scrapy (Version 1.6) [Computer software]. Retrieved from https://scrapy.org/